The public debate around AI and personal data revolves more around privacy concerns than opportunities. Perhaps rightly so, but AI also presents significant promise in protecting privacy. In this blog post, we list 4 use cases for AI in data protection.
Many AI algorithms are fueled by the personal data we share online. “Ignorance is bliss”, I think to myself while ticking another box without bothering to read the terms and conditions. If you, too, have given up hope on maintaining control over your personal data – hold on! There are many ways AI algorithms can be used to help protect your privacy.
“According to a survey by Brookings, 49% of people think AI will reduce privacy. Only 12% think it will have no effect, and a mere 5% think it may make it better. “
Zhiyuan Chen and Aryya Gangopadhyay, The Conversation 22.6.2020
It is true that AI systems, especially the ones based on deep learning, typically use lots of data. It is also true that preventing identity disclosure through traditional anonymization techniques is extremely difficult. Even a machine learning model itself can reveal too much about the data subjects included in the training set. This happens when the original training data, such as facial images, can be re-constructed by observing the model behavior (model inversion).
In the most serious case, some data points could be directly linked to you (identity disclosure). In other risk scenarios, the dataset could reveal something about you (attribute disclosure) or make it possible to infer whether you’re in it or not (membership disclosure). However, researchers have developed technical measures against all sorts of privacy disclosures.
According to Gartner, over 40% of privacy compliance technology will rely on AI systems by 2023.
The protective measures range from access control to the way the data is being processed and how the model learns from the data. In fact, there are options for addressing all 6 data protection principles listed in the European GDPR (General Data Protection Regulation). These measures can be used complementarily to keep personal data safe.
Next, I will list 4 examples.
- Improving data security through adaptive AI tools
One of the aims of data protection is to ensure that only authorized persons have access to the data. This is where data security enters the game. While password protection might be the only security measure you see as an end-user, cybersecurity professionals do a lot more to prevent data breaches. AI tools can be harnessed for automated surveillance and monitoring.
For example, machine learning models can learn what is typical user behavior and what is not. They can also be updated to handle new kinds of situations. Technically speaking, the tool’s ability to learn and adapt (“intelligence”) is achieved by fitting mathematical models and re-optimizing them. Any suspicious activity triggers other defenses to prevent a potential intrusion attack.
AI tools can also mitigate data security issues arising because of AI, for example when machine learning models are manipulated to reveal sensitive data. Adversarial machine learning is a relatively new research field that explores these kinds of threats. While the primary countermeasures point to technical implementation, one could, for example, apply another machine learning model to block suspicious data that is being fed to the model. - Making sense of the end-user license agreements
Artificial intelligence, more specifically natural language processing, has been used for reviewing and managing contracts. AI systems could act as privacy assistants, helping people to make sense of legal terminology and clauses.
Algorithms cannot replace human judgment, but they can speed up the process by highlighting the parts of the document that seem the most relevant and should be checked first. By doing this, they can also help users become more aware of the terms and conditions of everyday services, as well as their GDPR rights.
The Personalized Privacy Assistant Project, led by prof. Norman Sadeh from Carnegie Mellon University (US), goes further to envision semi-automated, personalized privacy settings and alerts.
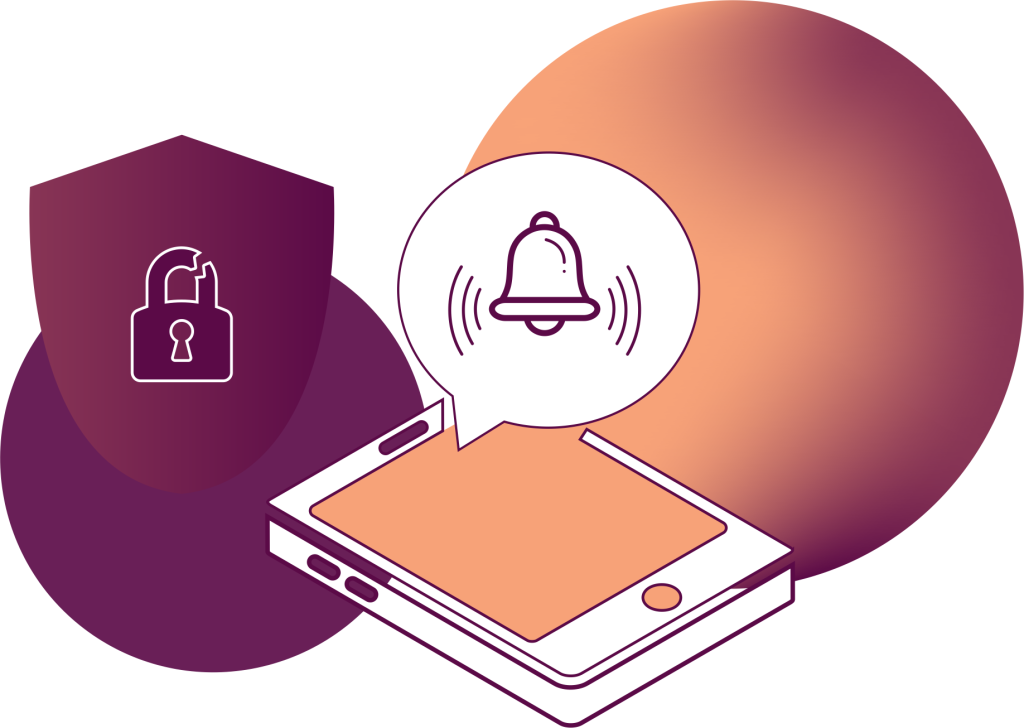
- Reducing the need to transfer and merge data
Modern machine learning methods don’t necessarily require all data to be stored in one place for the analysis to take place. Edge AI enables local processing with new, simplified AI tools that get the specific job done more efficiently.
In federated learning, the data never has to leave the device. Data can be distributed across different locations such as mobile phones. The aggregate model then combines the inputs from different locations to create a generalized model.
This approach represents a mixture of data minimization and confidentiality and security. - Reducing the need to process real data
Sometimes the best way to protect privacy is to critically evaluate, whether real data is needed at all. AI algorithms are becoming better and better at generating fake datasets that look and behave like real ones. For some purposes, this kind of synthetic data is a sufficient and much more privacy-friendly approach. Yet it is important to note that synthetic datasets are not private by default. If the generator uses real data as model input, additional privacy-preserving mechanisms must be applied to guarantee privacy.
For example, introducing a level of randomness to the synthesis effectively reduces risks. Anyone trying to extract personal data from the synthetic data or the generator (i.e., the machine learning model itself), would become confounded by the high level of uncertainty. With enough random noise added, it would be impossible to tell whether an attempt of privacy disclosure has been successful or not.
Project PRIVASA is focusing on the approaches 3 and 4, especially from the health data angle.
I suppose it’s fair to assume AI and big data analytics are here to stay, and there will always be people willing to misuse personal data. Proper safeguards, however, can shift the balance towards a more privacy-friendly future with AI.
Further reading:
https://theconversation.com/ai-could-help-solve-the-privacy-problems-it-has-created-130510
https://towardsdatascience.com/what-is-adversarial-machine-learning-dbe7110433d6
https://www.wired.com/story/edge-ai-appliances-privacy-at-home/